In the fast-paced world of technology and artificial intelligence, staying updated with the latest advancements is crucial. One such remarkable development in the field of neural networks is the emergence of Recurrent Residual Networks (RRNs). These networks have gained significant attention in recent years for their ability to overcome the limitations of traditional neural networks. In this article, we will delve into the fascinating world of RRNs, exploring their architecture, applications, and potential impact on various industries.
Introduction to RRNs
Recurrent Residual Networks (RRNs) are a cutting-edge innovation in the realm of neural networks, combining the strengths of Recurrent Neural Networks (RNNs) and Residual Networks (ResNets). They address the limitations of traditional neural networks by effectively capturing long-term dependencies in sequential data. Key points to understand about RRNs include:
- Hybrid Architecture: RRNs merge RNNs and ResNets, providing a powerful framework for sequential data analysis.
- Sequential Mastery: They excel at tasks involving sequences, such as natural language processing, speech recognition, and time series analysis.
- Gradient Flow: RRNs boast improved gradient flow, making them suitable for training deep architectures without the vanishing gradient problem.
- Enhanced Training: The combination of recurrent and residual connections simplifies training, leading to faster convergence and superior performance.
- Future Potential: RRNs continue to evolve and hold significant promise in shaping the future of artificial intelligence.
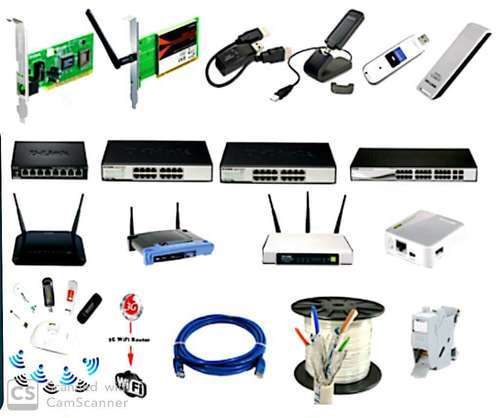
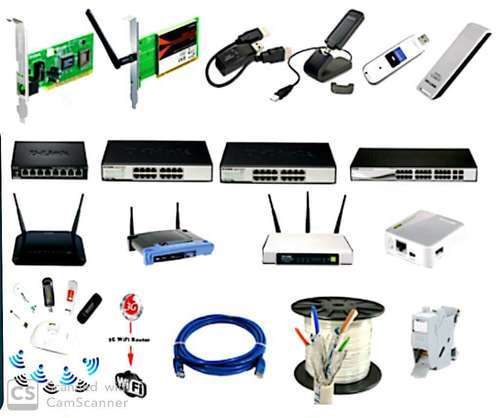
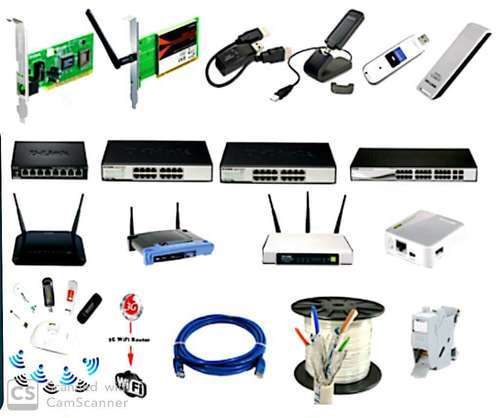
The Building Blocks of RRNs
1. Recurrent Neural Networks (RNNs) |
– Fundamental for handling sequential data |
– Maintain hidden states to capture context |
– Struggle with vanishing gradient problem in deep sequences |
2. Residual Networks (ResNets) |
– Introduce skip connections for improved gradient flow |
– Efficiently train deep neural networks |
– Solve the problem of vanishing gradients |
3. RRNs: The Hybrid Solution |
– Combine RNNs and ResNets to leverage their respective strengths |
– Utilize recurrent connections for sequential data |
– Benefit from residual connections for gradient stability |
4. Enhanced Training Process |
– Simplified training with the integration of both components |
– Faster convergence and improved performance |
– Address challenges posed by deep sequences |
How RRNs Work
- Recurrent Connections: RRNs incorporate recurrent connections, allowing them to efficiently process sequential data. These connections enable information to persist across time steps, making RRNs adept at capturing long-term dependencies in data sequences.
- Residual Connections: Building on the success of Residual Networks (ResNets), RRNs also feature residual connections. These connections ensure that gradients flow smoothly during training, enhancing the stability and effectiveness of the network.
- Combined Strengths: RRNs harness the strengths of both recurrent and residual connections. This unique combination enables them to excel in tasks involving sequential data, such as natural language processing, speech recognition, and time series analysis.
- Improved Gradient Flow: RRNs offer superior gradient flow compared to traditional neural networks, mitigating the vanishing gradient problem. This makes them well-suited for training deep architectures effectively.
- Efficient Training: The integration of recurrent and residual connections simplifies the training process, leading to faster convergence and improved model performance.
Advantages of RRNs
1. Improved Gradient Flow |
– Superior gradient flow, especially in deep architectures |
– Mitigates the vanishing gradient problem |
2. Enhanced Training Efficiency |
– Simplified training process due to combined connections |
– Faster convergence and shorter training times |
– Improved model performance |
3. Handling Long Sequences |
– Excel in tasks involving long data sequences, e.g., NLP |
– Capture complex dependencies and context effectively |
Applications of RRNs
- Natural Language Processing (NLP): RRNs have revolutionized NLP by effectively modeling context and relationships in text data. They excel in tasks like sentiment analysis, machine translation, and named entity recognition.
- Speech Recognition: In speech recognition, RRNs have significantly improved accuracy. They process audio data with long dependencies, making them ideal for transcribing spoken language and enabling voice assistants.
- Time Series Analysis: RRNs are highly proficient in analyzing time series data. They can predict trends, anomalies, and patterns in financial markets, weather forecasting, and stock price predictions.
- Handwriting Recognition: RRNs are used for recognizing handwritten characters and digits, enabling applications like digitizing handwritten documents and enhancing accessibility for visually impaired individuals.
- Video Analysis: RRNs are applied to video analysis, including action recognition and video captioning. They can effectively model temporal dependencies in video data.
- Genomic Sequence Analysis: In bioinformatics, RRNs are used to analyze DNA and protein sequences, aiding in gene prediction and functional annotation.
- Finance and Stock Market Prediction: RRNs are employed for modeling and predicting financial data, aiding investors and financial institutions in decision-making.
- Autonomous Vehicles: RRNs contribute to the perception and decision-making processes in autonomous vehicles, enhancing safety and navigation.
- Recommendation Systems: RRNs can be used to improve recommendation systems in e-commerce, entertainment, and content platforms, offering more personalized suggestions based on user behavior
Challenges and Limitations
1. Training Complexity |
– Computationally intensive, especially with deep architectures |
– Requires substantial computational resources |
2. Overfitting |
– Susceptible to overfitting, necessitating careful regularization |
– Can result in poor generalization and model performance |
3. Long Sequences |
– While RRNs excel at handling long sequences, excessively long sequences can still pose challenges in terms of memory and computation |
Future Prospects of RRNs
1. Ongoing Research and Refinement |
– Researchers continue to refine RRN architectures and training methods to improve performance and efficiency. |
2. Expanding Applications |
– RRNs are likely to find applications in new domains and industries as their capabilities become more recognized. |
3. AI in Healthcare |
– RRNs hold potential in medical image analysis, disease prediction, and drug discovery, contributing to advancements in healthcare. |
4. Enhanced Natural Language Understanding |
– RRNs will play a significant role in enhancing conversational AI and chatbots, leading to more human-like interactions. |
5. Autonomous Systems |
– RRNs will continue to be integral in autonomous systems, improving robotics, self-driving cars, and drones. |
Conclusion
Recurrent Residual Networks (RRNs) represent a pivotal advancement in the field of neural networks. Their ability to seamlessly merge the strengths of Recurrent Neural Networks (RNNs) and Residual Networks (ResNets) has opened up new avenues in the world of artificial intelligence. Here are the key takeaways:
-
- RRNs offer improved gradient flow, mitigating the challenges posed by vanishing gradients in deep architectures.
- Their training efficiency leads to faster convergence and enhanced model performance.
- RRNs excel in handling long sequences, making them invaluable in fields like natural language processing and speech recognition.
- These networks have found diverse applications across industries, from healthcare to autonomous systems.
Frequently Asked Questions (FAQs)
RRNs, or Recurrent Residual Networks, combine the strengths of Recurrent Neural Networks (RNNs) and Residual Networks (ResNets). They excel in capturing long-term dependencies in sequential data, which is a limitation in traditional neural networks.
While RRNs are primarily designed for sequential data, they can be adapted for image recognition tasks by preprocessing images into sequences. However, for standard image recognition tasks, convolutional neural networks (CNNs) are often preferred.
Yes, RRNs can be computationally intensive, especially when dealing with deep architectures and long sequences. Training RRNs may require substantial computational resources, including powerful GPUs.
Businesses can leverage RRNs for tasks like sentiment analysis, demand forecasting, and customer behavior prediction. These networks can provide valuable insights by capturing complex patterns in data.
To delve deeper into RRNs, you can find numerous online courses, tutorials, and research papers available on platforms like Coursera, GitHub, and arXiv. These resources offer a comprehensive understanding of RRNs and their applications.