Deep learning is a sub-field of machine learning and an aspect of artificial intelligence. To understand this more easily, let’s understand that it is meant to imitate the learning approach that humans use to acquire certain types of knowledge. This is different from machine learning, often people get confused about this and machine learning. Deep learning uses an indexed algorithm whereas machine learning uses a linear algorithm. To understand this more accurately, consider this example that if a child is identified with a flower, he will ask again and again, is this a flower? For him every colourful thing will be a flower, he will slowly sort things according to the flowers and slowly he will come to know about the flower. It develops over time.
Use of Deep Learning
Deep learning is being used very fast in today’s time, almost every major company is using it, or wants to. Some of its recent big uses have been made by the big companies of the phone, which include these things.
Image Recognition – It means to recognize a picture, it can often be easily seen on mobile phones.
Speech Recognition – Its job is to recognize the voice of the people.
Translator – Its job is to convert one language into another language. Many more examples of deep learning can also be found.
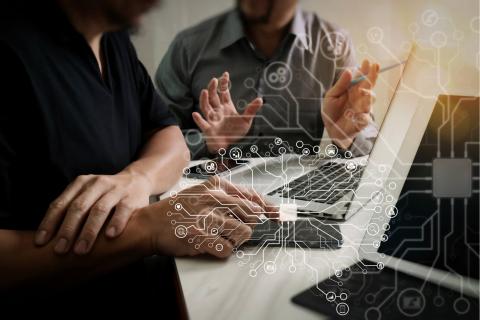
Limitations of Deep Learning
The biggest limitation of deep learning is that it learns only by observation. This means that it knows only that much of the data it is given. If someone does not have a large amount of data available then it will not work in that condition. If the data is collected in a biased manner, the result will be tilted more towards either side. That is, whatever you give it, it will learn from it and will give you results.
Prerequisites
- Programming is the fundamental requirement of deep learning
- Statistics refer to the study of using data and its visualization
- Calculus forms the basis for many machine learning algorithms.
- Linear Algebra.
- Probability.
- Data Science.
Popular Courses | Duration | Criteria |
Certified programs | 1year or less | 12th grade with computer application and programming language and B. Sc. or B. Tech in Technology, Mathematics, Engineering, CSE. |
Diploma and PG diploma courses. | 1-2 years | Undergraduate degree in Science, Computer Application, Mathematics or Engineering with 50% marks. |
Bachelor Courses. | 3-4years | 12th or Higher Secondary with Physics, Mathematics or Computer Science as compulsory subjects with 50% aggregate marks. |
Master Courses. | 2 years | Graduation in CSE in Deep Learning, Machine Learning from a recognized university with 50% marks. |
Doctorate Courses. | 3-4 years | M.Sc. or M.Tech in CSE (Computer Science Engineering) with a minimum of 50% marks from a recognized university. |
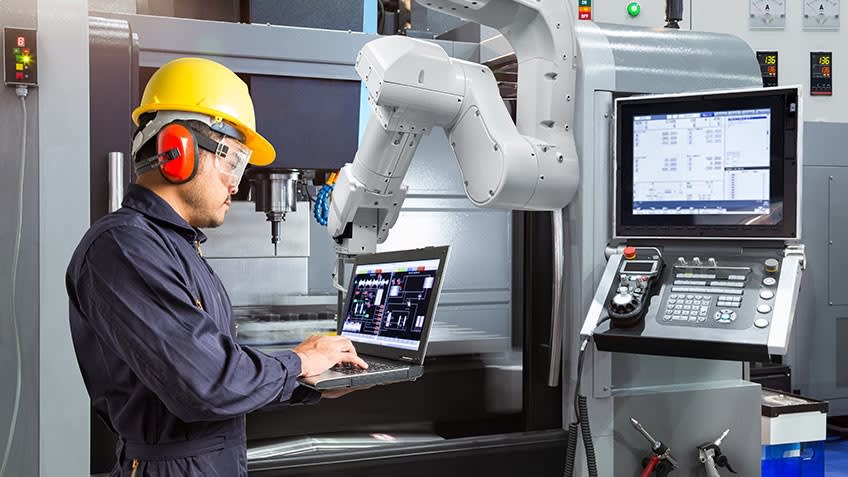
Top Collages
- Stanford University, California
- Massachusetts Institute of Technology, Cambridge
- National Research University- Higher School of Economics
- IIT Bombay Mumbai, Maharashtra
- IIT Roorkee, Uttarakhand
- Gautam University Visakhapatnam, Hyderabad
- NIELIT, Calicut
- K. Gujral Punjab Technical University, Jalandhar, Punjab.
- Panipat Institute of Engineering & Technology
- Silver Oak University, Ahmedabad
- University of Hyderabad
Top online courses
- Deep Learning A-Z™: Hands-On Artificial Neural Networks (Coursera)
- Complete guide to tensor flow for deep learning with python (Coursera)
- Data Science: Deep Learning and neural networks in python (Coursera)
- Machine Learning Certification by Stanford University (Coursera)
- Deep Learning Certification by deeplearning.ai (Coursera)
- Deep Learning Nanodegree Program (Udacity)
- Professional Certificate in Deep Learning by IBM (edX)
- Machine Learning Specialization by University of Washington (Coursera)
Salary insight
The average salary for “deep learning” ranges from $77,562 per year for Research Scientist to $135,255 per year for Machine Learning Engineer.
- Certificate: INR 4-5 LPA
- Diploma & PG Diploma: INR 6-9 LPA
- UG: INR 6-12 LPA
- PG: INR 8-13 LPA
- Doctorate: INR 10-19 LPA
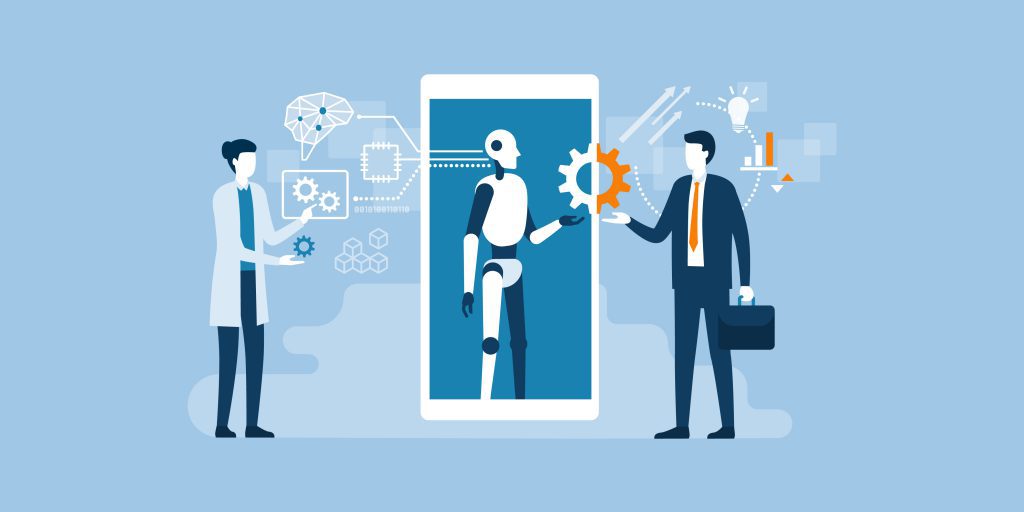
Future Scope
Deep Learning (DL) has a vast scope in future. The reason for it is that DL doesn’t require any kind of feature engineering. It extracts the features from the data itself instead of us giving it the features after extracting it from the data. Features are learned by the model itself, therefore it has a better probability of producing a model which is more generalized than the feature engineered models. With the recent development in DL, more tasks including Natural Language Processing, Language Translation, Automatic Speech Recognition, Multi-Label Image Recognition/Classification, Language generation among others, AI has grown stronger in the past 8 years with DL than it has developed in 20 years before that. So, if you are looking for a future in Deep Learning, you are looking in the right direction.
Jobs
- Software Engineer
- Research Analyst
- Data Analyst
- Data Scientist
- Data Engineer
- Neuroinformatics
- Bioinformatician
- Image Recognition
- Software Developer
- Research Scientist
- Research Fellow
- Instructor for Deep Learning
- Applied Scientist
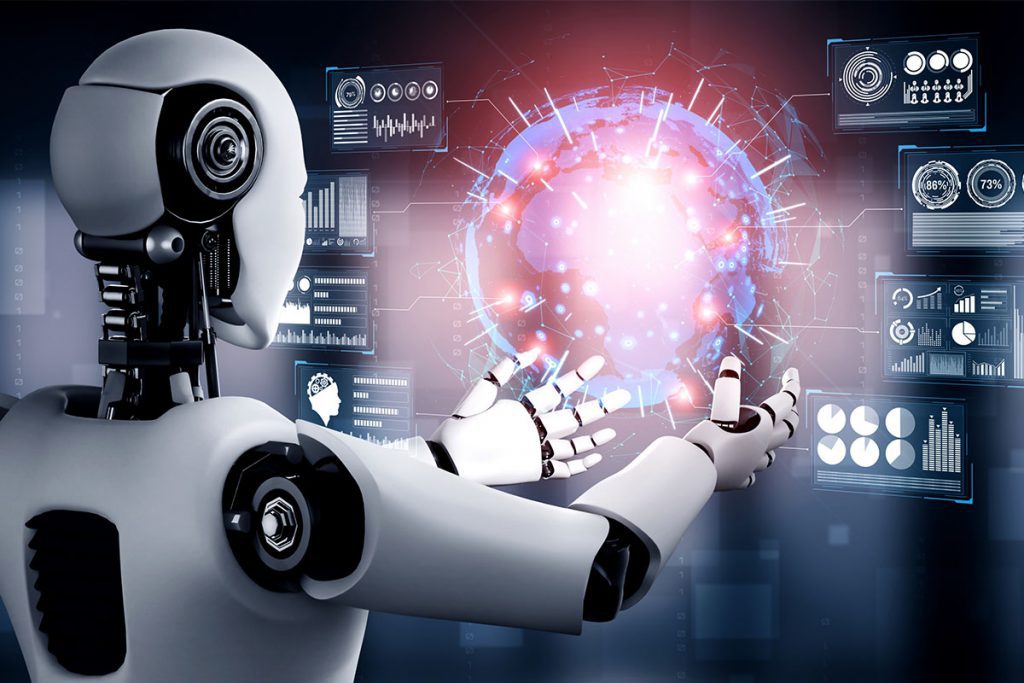
– Aakansha Jumle